Introduction to Shotgun Metagenomics, from Sampling to Data Analysis
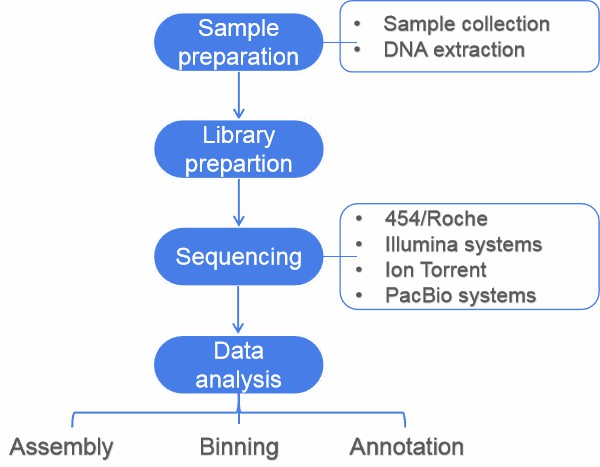
What Is Shotgun Metagenomics
Metagenomics is the science that applies high-throughput sequencing technologies and bioinformatics tools to directly obtain the genetic content of a microbial community without the need to isolate and culture the individual microbial species. Metagenomics enables researches not only to study the functional gene composition of microbial communities but also to conduct evolutionary research. Metagenomics has been used to identify novel biocatalysts or enzymes and generate novel hypotheses of microbial function, which is a powerful and practical tool. Compared to 16S/18S/ITS amplicon sequencing, metagenomics can provide more information about functional potential of microbial communities and whole-genome sequences. The rapid development and substantial cost decrease in high-throughput sequencing have dramatically promoted the development of shotgun metagenomic sequencing.
This article gives an overview of metagnomics, from sampling to data analysis. A typical metagenomics project involves sample preparation, sequencing, and data analysis (including assembly, binning, annotation, statistical analysis, and data submission).
Figure 1. Flow diagram of a typical metagenome project.
How Dose Shotgun Metagenomics Work
Sample preparation
Sample preparation generally involves two steps, sample collection and DNA extraction, both of which can affect quality ad accuracy of metagenomic experiments. Commercial kits are available for sample collection and DNA isolation. Its key objectives are to collect enough microbial biomass for sequencing and to minimize contamination. When working with low biomass samples, ultraclean reagents and "blank" sequencing controls should be used to minimize less "real" signals.
Library preparation and sequencing
Common high-throughput sequencing platforms include Illumina systems, Roche 454, Ion Torrent instruments, and PacBio SMRT systems.
Frey et al. (2014) assessed the ability of three next generation sequencing (NGS) platforms (Illumina MiSeq, Roche 454 Titanium, and Ion Torrent PGM) to identify a low-titer pathogen (viral or bacterial) in a clinically relevant blood sample. They found that Ion Torrent PGM and Illumina platforms perform better in identification of scarce microbial species, and for bacterial samples, only the MiSeq platform could provide reads that were unambiguously classified as originating from Bacillus anthracis.
The Illumina platform has become dominant for shotgun metagenomics sequencing due to its very high outputs (up to 1.5Tb per run), high accuracy (error rate of between 0.1-1%), and wide availability. Ion Torrent instruments and PacBio SMRT instruments are becoming tough competitors in the field. The Illumina platforms mainly differ in total output and maximum read length. The Illumina HiSeq 2500 (2x250 nt, 180 Gb output or 2x125 nt, 1Tb output) is a classical choice for metagenomics. Newer HiSeq 3000 and 4000 systems increase the throughput of a run but are limited to read length (150 nt). The MiSeq instruments only generate up to 15Gb in 2x300 mode but are still useful for single marker gene microbiome studies, or a limited number of samples.
Pacific Biosciences (PacBio) instruments, based on single-molecule, real-time (SMRT) detection in zero-mode waveguide wells, provide much greater read lengths (average read lengths up to 30 kb) than NGS instruments. Short-read sequencing (i.e. NGS) has limited ability to assemble complex or low-coverage regions, while long-read metagenomic sequencing by PacBio SMRT sequencing is able to reconstruct a high-quality and closed genome of a previously uncharacterized microbial species from metagenomic samples.
Data analysis
- Assembly
If the research aims at obtaining full-length CDS or recovering microbial genomes, then assembly need to be performed to generate longer genomic contigs. Assembly can be divided into two strategies: reference-based assembly and de novo assembly. Reference-based assembly is fast and accurate, if the metagenomic dataset includes sequences where closely related reference genomes are available. Reference-based assembly can be performed with software packages such as Newbler, AMOS, MIRA. De novo assembly requires larger computational resources. De Bruijin graph approach is the most popular metagenome de novo assembly method.
If the research aims at taxonomic profiling, there is no need for assembly and binning. Assembly-free metagenomic profiling can mitigate assembly problems, and make it possible to identify low-abundance species that cannot be assembled de novo. The approach is limited because previously uncharacterized microorganisms are difficult to profile, but the number of reference genomes is increasing rapidly.
- Binning
Metagenome assemblies are only fragmented contigs. We do not know contig derives from which genome. We do not even know how many species there are. Binning is the process of grouping contigs into species. There are two strategies for binning, including compositional-based and similarity-based methods. The examples of compositional-based binning algorithms include S-GSOM, Phylopythia, PCAHIER, and TACAO. Similarity-based algorithms include IMG/M, MG-RAST, MEGAN, CARMA, SOrt-ITEMS, MetaWatt, SCIMM, and MetaPhyler. Some algorithms consider both composition and similarity, such as PhymmBL and MetaCluster.
- Annotation
The annotation has two steps, gene identification and functional annotation. Databases that contain combinations of manually annotated and computationally predicted proteins families, can be used for genes and metabolic pathway from metagenomes. Common databases and tools are concluded in the following table.
Table 1. Common databases and tools for annotation of metagenomic data.
Databases/Tools | Details |
KEGG | KEGG is a database resource used to understand functions and utilities of the biological system. |
UniProt | UniProt provides a repository of metagenomic sequence data and allows you to view taxonomic and functional analyses. |
TIGRFAM | TIGRFAMs is a database of protein family definitions. |
eggNOG | eggNOG is used for identification of orthologous gene groups and function annotation. Other databases of orthologous gene groups include KEGG, COG, M5NR, and Metacyc. |
SILVA | SILVA is an on-line resource for quality checked and aligned ribosomal RNA sequence data. |
Greengenes | Greengenes is a combination of a chimera-checked 16S rRNA gene database and tools. |
RDP | Ribosomal Database Project (RDP) includes aligned and annotated rRNA gene sequence data and tools. |
HUMAnN pipeline | HUMAnN is a pipeline for accurately determining the presence/absence and abundance of microbial pathways from metagenomic data. |
CAZy | CAZY (Carbohydrate-Active enZYmes) database can be used for prediction of genes coding for carbohydrate-active enzyme and correlation analysis. |
CARD | The comprehensive Antibiotic Resistance Database (CARD) can be used for prediction of resistance genes and correlation analysis. |
MG-RAST | MG-RAST is an open source web application server for phylogenetic and functional analysis of metagenomes. |
Conclusion
Since whole DNA sequencing of environmental sample was first performed by teams led by Banfield and Venter in 2004, shotgun metagenomics has become an indispensable tool for the study of microbial communities. The decreased cost of sequencing and the development of computational methods have promoted the widespread adaption of metagenomics.
The realm of metagenomics brings forth numerous advantages, although it is not without certain drawbacks. A significant advantage includes the ability to bypass the requirements for microbial cultivation, allowing for direct extraction and analysis of microbial DNA from environmental samples. This successfully averts the limitations and biases intrinsic to traditional cultivation methods. Another strength of metagenomics lies in its comprehensiveness, enabling thorough and swift insights into the composition and functional potentialities of microbial communities. This includes less cultivable microorganisms and genes with yet unknown functions. Furthermore, it provides high-resolution analysis capabilities to reveal microbial diversity, structure, and functionalities from an individual to a community level. Additionally, metagenomics aids in the discovery of new microbial species and functional genes, opening up the possibilities for novel utilizations of microbial resources. Lastly, metagenomics manifests broad prospects across fields such as ecology, biomedicine, industry, and environment, presenting an effective means to tackle diverse sets of problems.
While metagenomics offers remarkable insights, it also presents a number of inherent challenges. Primarily, the sheer scale and intricacy of the burgeoning data is a significant challenge to its interpretation and analysis, necessitating the application of sophisticated, specialized methodologies and techniques. The data analysis demands substantial computational resources, including professional-grade software tools, which frequently incur significant expense and time commitments. Furthermore, the extraction process of environmental samples, replete with their inherent complexity and potential for contamination, can introduce extraneous noise into the data. This noise can, in turn, compromise the precision of the outcomes. The bioinformatics landscape, with its assortment of trials, including sequence assembly, functional annotation, and species composition analysis, underscores the constant requisite for the refinement and enhancement of the workflow.
Despite these challenges, the field of metagenomics nonetheless presents immense potential. As technologies related to sequencing continually advance and associated costs decrease, the application of shotgun metagenomics sequencing is predicted to become increasingly pervasive. Simultaneously, we expect that the evolution of bioinformatics will yield more efficient and more accurate tools and algorithms for data analysis. This, in turn, would facilitate an enhanced interpretation and utilization of sequencing data. Moreover, the amalgamation of metagenomic data with other omics data sets, in a comprehensive analysis, promises to reveal more profound insights into the functionality and interplay within microbial communities. In the landscape of medical microbiome research, metagenomics could serve as a revolutionary tool for precision medicine. It seeks to open fresh avenues for the enhanced diagnosis, treatment, and prevention of diseases. Looking towards environmental conservation and biotechnology, the anticipated future of metagenomics hosts a range of more efficacious approaches for gauging environmental impacts, exploiting biological resources, and conducting bioprocess engineering.
References:
- Faust K, Lahti L, Gonze D, et al. Metagenomics meets time series analysis: unraveling microbial community dynamics. Current opinion in microbiology, 2015, 25: 56-66.
- Frey K G, Herrera-Galeano J E, Redden C L, et al. Comparison of three next-generation sequencing platforms for metagenomic sequencing and identification of pathogens in blood. BMC genomics, 2014, 15(1): 96.